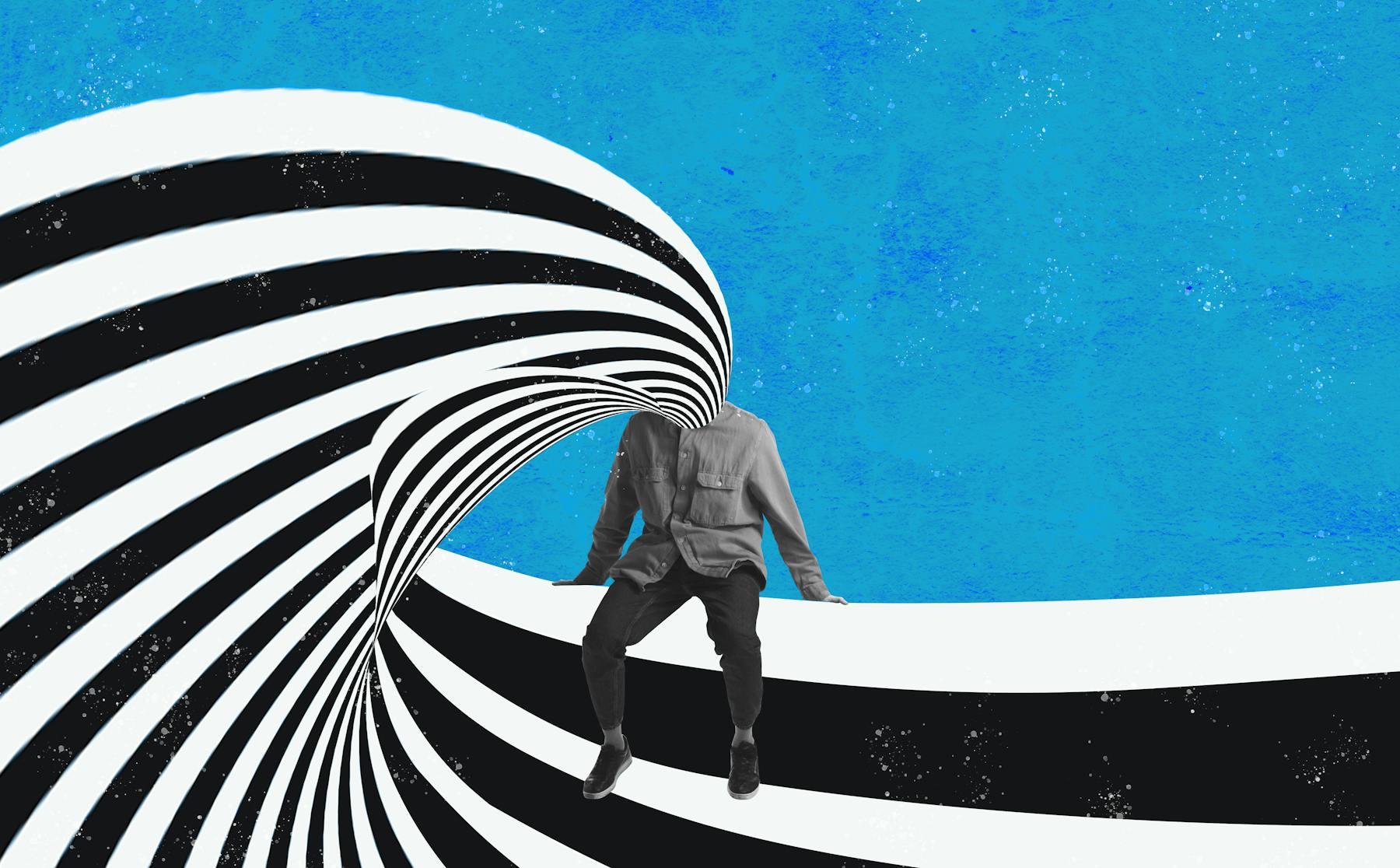
Synthetic Data (otherwise known as making things up)
CEO David Alterman takes a moment to reflect on the rise of synthetic data in market research
Our professional lives are being transformed by generative AI, apparently. The launch of Chat GPT just 12 months ago and a range of competing products has suddenly brought the power of artificial intelligence into the mainstream of marketing, research and analytics.
Market research companies are tripping over each other to launch their AI enhanced research services; quicker, cheaper, smarter with the added value of minimising the amount of human interaction required.
The thing is that people make really bad survey respondents or group participants; they are illogical, irrational and inconsistent. They contradict themselves, switch off randomly, show remarkably little passion for some of the brands they buy, and seem incapable of answering simple questions – like why did you buy Brand X, visit Place Y, decide to do Z?
Finally now a range of artificial intelligence applications are available that can solve this problem once and for all. No more rubbish organic data – welcome to the world of synthetic data – complete, consistent and logical.
Synthetic data is of course nothing new. In the 90s I worked for a research company in New Zealand with some clever people who were at the front edge of data fusion in market research – taking two big datasets – one which captured TV ratings data, the other a range of purchase, usage and other media consumption data – and finding a number of hooks where we could look for respondent twins who matched across the two databases. Once we found them we could fuse the TV viewing data from one twin onto the other twin and vice versa. Ta Da. Now we had one integrated database with TV viewing, purchasing and media data all ascribed to the same individual.
Over the years as the techniques became more sophisticated and the computers more powerful, the ability to ascribe data to individuals who neglected to supply it became ever more prevalent. Some of the most public and high profile applications relate to modelling voting intention using MRP – taking large national samples and then looking at underlying patterns in the data to provide constituency level predictions.
These mass market applications can make sense – if creative use of these tools can provide some guidance on targeting, media buying, macro behavioural trends then that is great.
Where I am struggling a bit is for assessing creative response and qualitative insight whether from qual samples or quant surveys. These human inconsistencies that are ironed out so efficiently provide clean credible data but does it reflect messy reality? Does the process squeeze the life out of the organic data and hide a truth that could make a difference?
In our increasingly commoditised world where brand differentiation is at such a premium don’t we need a dose of reality to find the difference that could just make a difference?